Every big question we ask about branding, performance, budgets, and goals is about the same thing: proving beyond a doubt that marketing works.
Or which bits of it work and which don’t?
And the right tool for the job is incrementality analysis.
As Chris Karvinen from Sellforte wrote;
“I click, therefore the ad is working” -philosophy isn’t cutting it anymore in 2023. Understanding incrementality plays a key role in improving marketing effectiveness.”
What is Incrementality analysis?
Incrementality means additional growth that can be directly linked to marketing above and beyond the existing brand equity or physical availability.
Going back to Chris;
“Models that measure incrementality, such as Marketing Mix Modeling, start by estimating what is the baseline, so what would’ve happened without any marketing activities.”
Incrementality measures the impact of each marketing activity independently.
As this post from Remerge explains, “Rather than relying on the correlation of events, it uses a Randomized Controlled Trial (RCT) to observe differences in behavior (conversion rate, revenue per user, etc) between a ‘treatment group’ that is targeted by ads and a ‘control group’ that is not targeted by ads.”
The fastest way to calculate incrementality is to find the conversion difference between the test group and the control group.
(Test Group Conversion Rate – Control Group Conversion Rate) / (Test Group Conversion Rate) = Incrementality
Here you can find an example of this from Marketing Evolution.
Incrementality analysis solves the big problem with attribution modeling.
The same Remerge article explains, “all [attribution] models rely on timely correlation of events per user (“first this happened, then that happened for the same user”) to derive the concept of attribution, which in fact is often being perceived as causality (‘the user clicked on that ad, so we attribute this revenue to that ad” sounds very similar to “that ad caused that revenue’).”
That’s the big problem with attribution modeling: it looks at clicks and skips over all other important factors that drive sales such as brand awareness, seasonality, promotions, or holidays.
I think this image from Chris’s article, describes the difference between attribution and incrementality better than anything I can do with words.
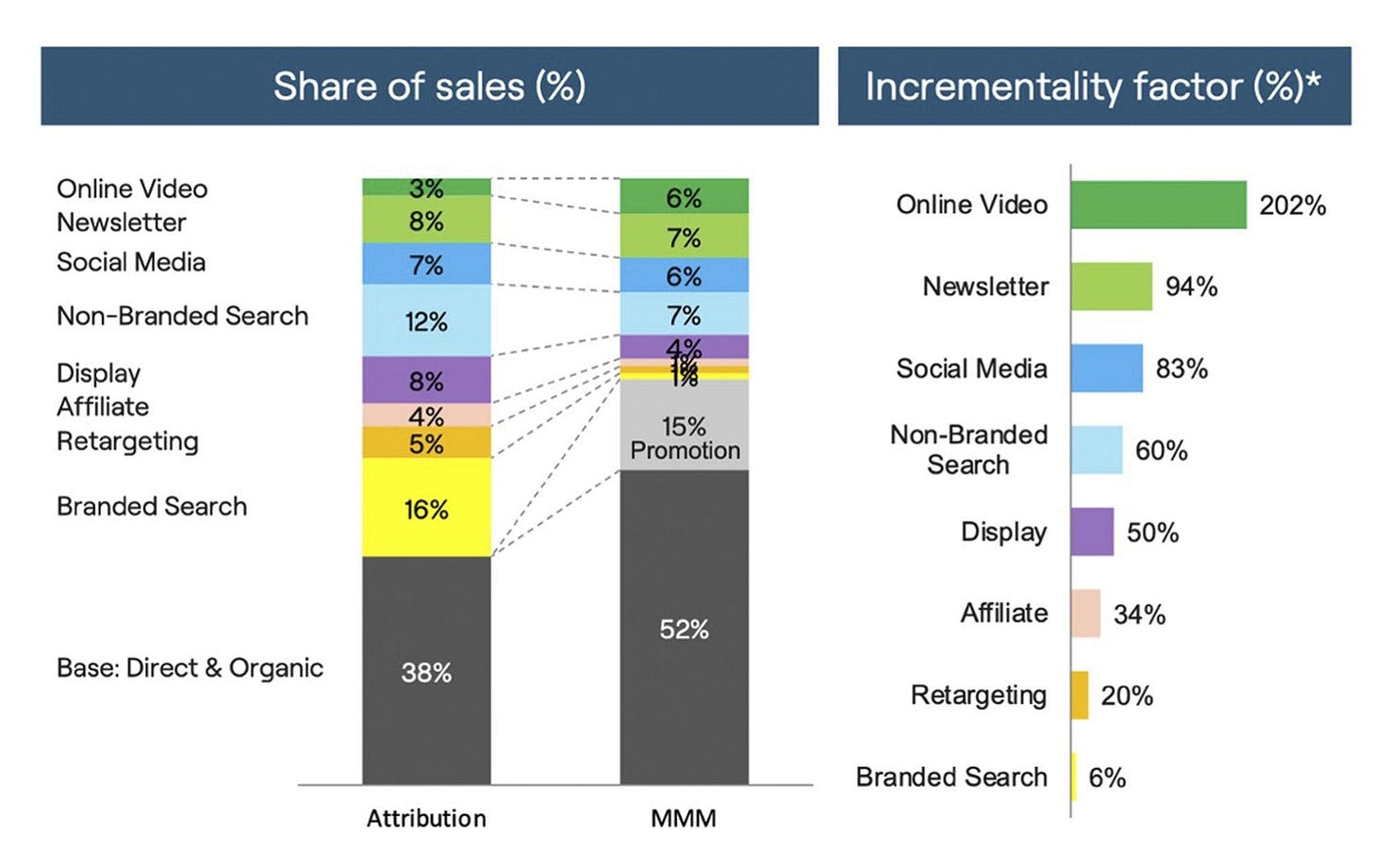
Incrementality measures growth that can be directly attributed to specific marketing activity above and beyond the existing brand equity or distribution.
But there’s more to it.
As Avinash Kaushik recommends, “Regardless of your business or budget size, you need to understand the concept of incrementality. I mean, really get it. In addition to the benefits to your marketing, it just might get you a raise and a promotion.” (emphasis added)
Really understanding Incrementality
The most common type of incrementality analysis is a holdout test.
A holdout test involves dividing your target audience into two groups: a test group and a control group. Then, you target the test group with your chosen marketing strategy, while leaving the control group alone.
Once both groups, those exposed to your ad and those who weren’t, have had a chance to see the different strategies, you can compare their engagement levels to see if the strategy actually made a difference.
Holdout tests can be scaled to testing a channel or even a marketing strategy.
Separating 'exposed and unexposed users' properly and without bias us where things get complicated.
And this is where incrementality analyses become more complicated than measuring clicks.
Referring to Remerge once more,
“The disadvantages of incrementality lie in the complexity of this methodology. On the surface, incrementality measurement might seem like an easy concept: randomly split the population, only show ads to one group, then observe the results. Though this is just the tip of an iceberg - to apply it successfully one requires much more detailed knowledge in the areas of different methodologies, statistics, parameters affecting noise, analysis framework, typical biases, and flaws. Another problem with incrementality is that it requires much more data (sample size in unique users) to determine statistically significant results. Therefore it is difficult to apply it on a granular level: per segment/per campaign results.” (Emphasis added)
Further complexity comes from avoiding selection bias (who goes into each group) and the challenge of treating ‘unexposed users’ who add to the complexity but practically don’t affect either cost or conversions.
Then there are non-marketing activities, as Optimine points out, “Accounting for non-marketing factors (examples listed below) is critical to understanding how effective your marketing campaigns are. You’ll misstate the value of ads if you aren’t accounting for these elements.”
Incrementality analysis appreciates the complexities of the real world without limiting your marketing to clicks.
As Trevor Testwuide explains, attribution doesn’t work for channels “where ad impressions are difficult to map and measure such as walled garden social channels including Facebook, Snap and Pinterest, or even TV and direct mail. For most channels or platforms, MTA [Multi-touch attribution] cannot measure views or impressions, so you’re essentially just measuring clicks.”
Incrementality analysis uses different statistical techniques to fit you data and remove bias.
Where attribution only looks at instances where an action took place. Only measuring the instances where you succeeded and looking past every other instance where you didn’t is the definition of bias.
Once again referring to Remerge;
“Intent-to-Treat and Ghost Bids are examples of two techniques that include ‘exposed’ and ‘unexposed’ users in the treatment group (to a different degree) and don’t provide the information which users in the control group ‘would have been exposed’.”
And this is where incrementality takes a leap over attribution as the article goes onto explain, “the revenues of the unexposed users are not simply ‘attributed’ to the campaign - they are used as a sum with the revenues of exposed users to then later be compared to the revenues of the control group (potentially including scaling).”
Incrementality avoids the ‘success bias' by treating people who saw your ad, those who did not, and those who might have seen it the same way.
This is a good place to stop for today.
Incrementality analysis is the promise of data-driven marketing. And I've only scratched the surface.
There's so much more to explore and I'm eager to dive deeper into it next week with marginal costs and cannibalisation.
Have the best week!
Aliyar